Built for V2X Communication
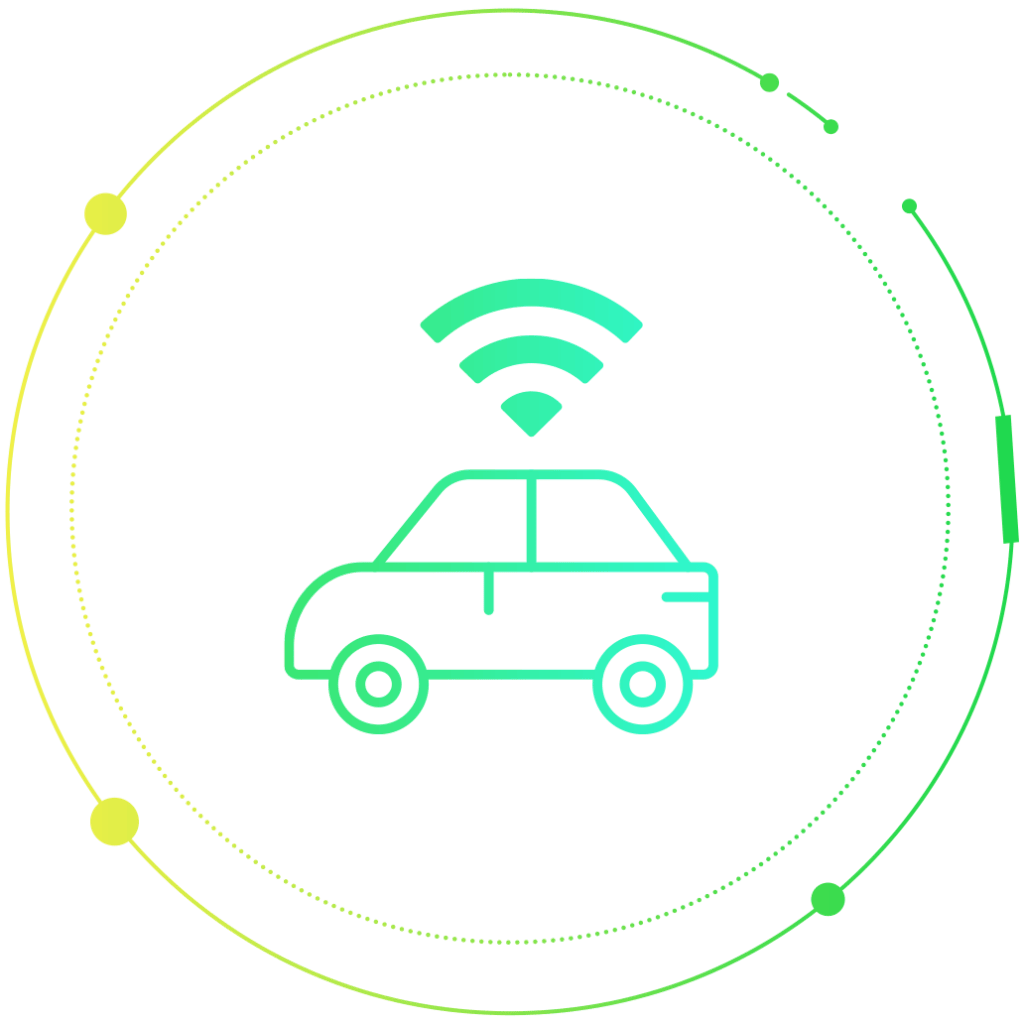
TDengine securely connects to MQTT data sources, making digital transformation easier than ever.
Deploy TDengine Enterprise on-premises, in a private cloud, or use the fully managed TDengine Cloud.
TDengine is purpose-built for handling massive datasets for fleet management or autonomous driving; store, process, and monitor up to petabytes of data per day.
Solution Architecture
Deploying TDengine reduces system complexity and costs for vehicle-to-everything (V2X) communications
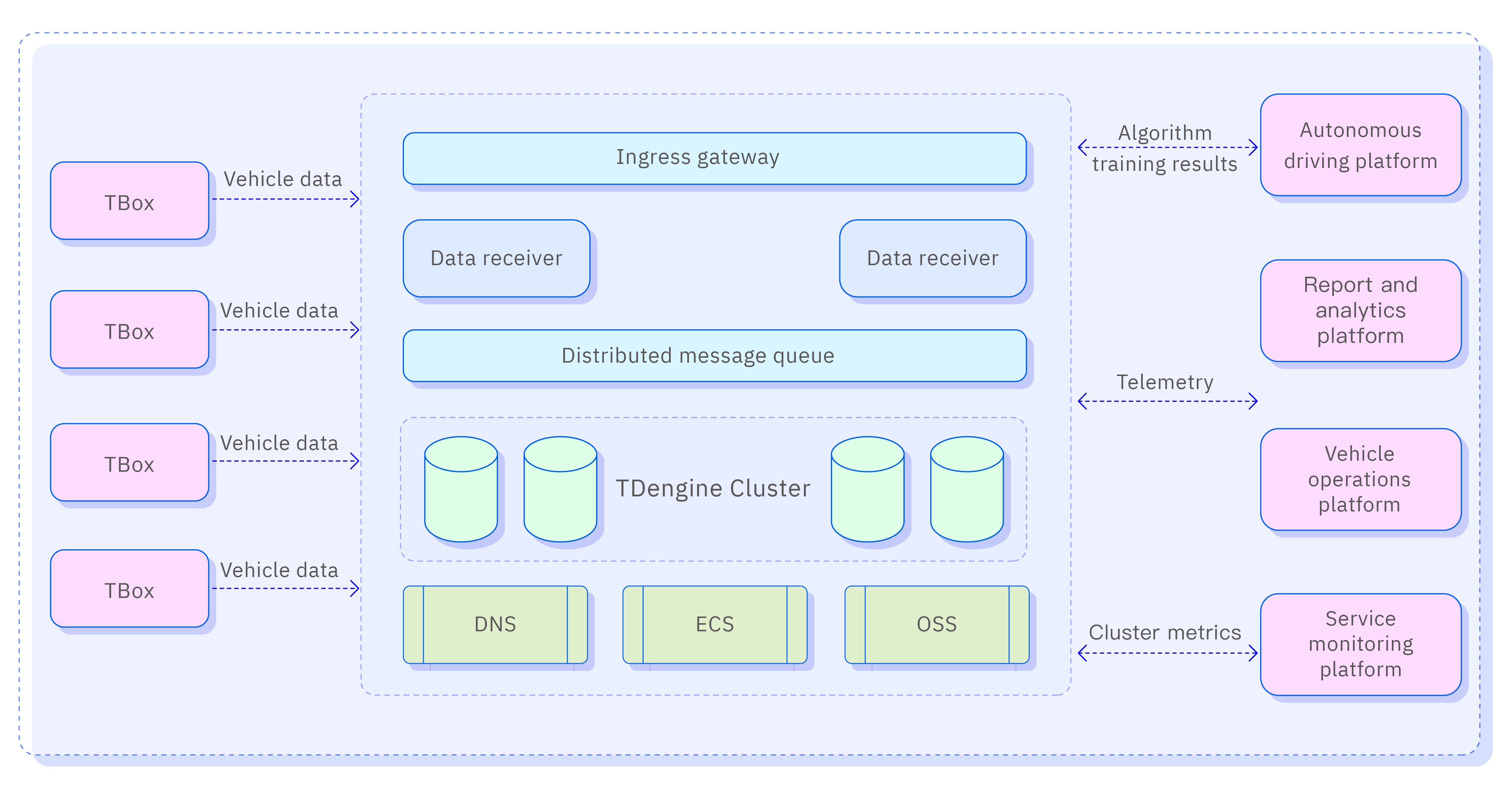
How TDengine Benefits You
01
Query response in milliseconds, even up to a million vehicles with a thousand collectors each
02
Server costs reduced by up to 70% and storage costs by up to 50% compared with traditional database solutions
03
Simplified system architecture with built-in stream processing, caching, and data subscription components
Case Study: DJI Automotive
"In DJI Automotive's current cloud platform, the application of TDengine not only saves storage costs and development and learning costs, but also shows good write and read performance, meeting the processing needs of massive time series data on the intelligent driving cloud platform. On the query side, whether it's a query that selects specific data or a lightweight query, it returns data in milliseconds."
Background
DJI Automotive, a subsidiary of industry-leading drone manufacturer DJI, announced its entrance into the field of autonomous driving last April. Considering the high frequency at which its smart cars report data back to the system, DJI Automotive quickly realized that to process this massive set of autonomous driving data, their data historian would require the following capabilities:
- Store tens of millions of records per table in order to contain the massive amounts of autonomous driving data that DJI Automotive will generate.
- Quickly filter this huge data set by means of aggregate functions.
- Support clustering for high availability and backup functionality.
- Use an industry-standard query language so that technical personnel can use the system without special training.
With its relatively recent entrance into the market, DJI Automotive was not particularly burdened by legacy data, and all database solutions were on the table.
Solution
In the TDengine solution, vehicles send their information to the MQTT broker. The application also sends commands to vehicles through the MQTT broker. The MQTT streams sent between devices and the cloud and between the cloud and the application are forwarded to a Kafka message queue, from which they are consumed by the business system. The system resolves these messages into various items that are stored in TDengine.
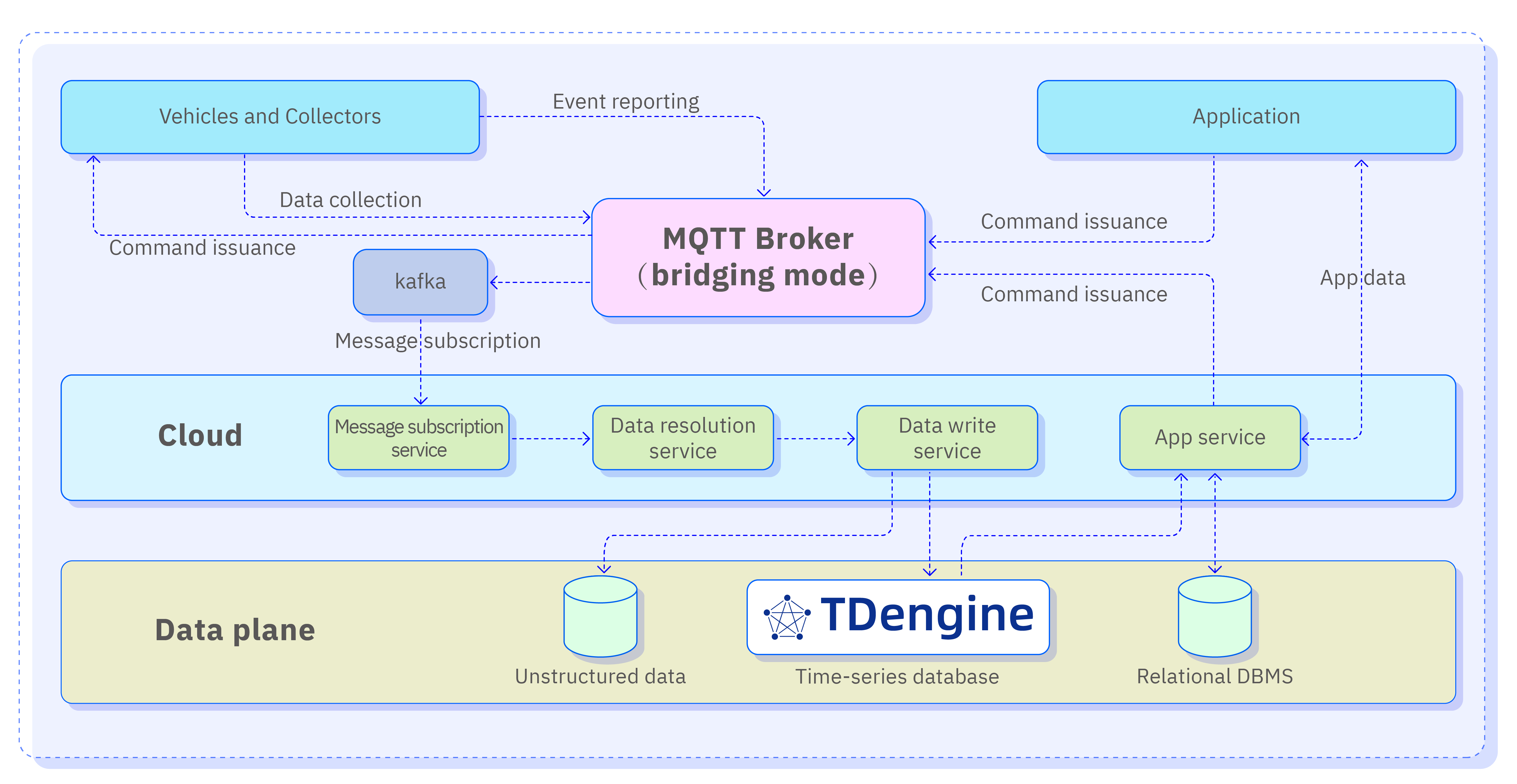