An in-depth look at the performance of key time-series databases
For a quick overview, see our infographic or watch a video
The sheer volume and complex nature of time-series data has given rise to the purpose-built time series database (TSDB). While performance is crucial for all databases, the scale and complexity of time-series data makes performance, precision, and scalability especially important.
The performance of your TSDB doesn’t just impact your ability to ingest, store, and analyze large amounts of data; it directly affects your total cost of ownership (TCO). Better ingestion rates, query response times and compression ratios mean your system consumes fewer resources to process the same amount of data. To demonstrate TDengine’s robust performance, we evaluated the platform against two key players in this space: InfluxDB and TimescaleDB.
The findings clearly show that TDengine offers significant advantages over both InfluxDB and TimescaleDB in terms of data ingestion, compression, and query performance. In addition, TDengine uses fewer server-side CPU resources than either competing time series DB to process identical datasets.
Objective Evaluation via the Time Series Benchmark Suite
To ensure an even playing field, we used the open source Time Series Benchmark Suite (TSBS) framework and ran the tests on identical systems in AWS. TSBS is designed for objective database evaluations and generates datasets for a range of recommended ingestion and query scenarios. TSBS is used by other database providers, including VictoriaMetrics and Timescale, to perform evaluations similar to the one described here.
TSBS is an open and independent framework, meaning the test procedures and datasets generated aren’t designed to benefit any database platform, and allows anyone to conduct an objective evaluation.
This evaluation applied the TSBS DevOps dataset, which includes small and large-scale scenarios, each with ten metrics, ten tags, and a microsecond-precision timestamp. For detailed information, see the official TSBS repository in GitHub.
Performance Comparison
Ingestion Performance
Time series databases need to ingest massive amounts of data, and TDengine achieves the fastest ingestion speeds across all TSBS scenarios, ranging from 1.5 to 10.6 times the speed of the other products.
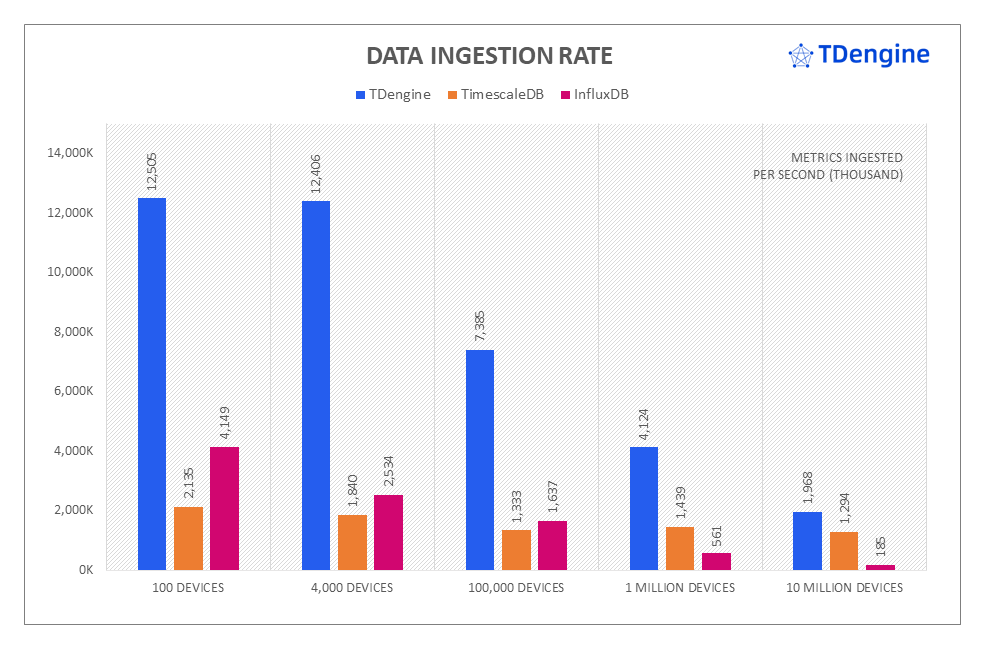
Resource Consumption
In addition, TDengine uses less processing power than InfluxDB or TimescaleDB to ingest the datasets. At its peak, InfluxDB’s CPU usage exceeds 90% during the ingestion process, while TDengine remains under 15%. Although TimescaleDB used a similar amount of CPU resources to TDengine, it spent far more time to compress and order the data after writing it to the database.
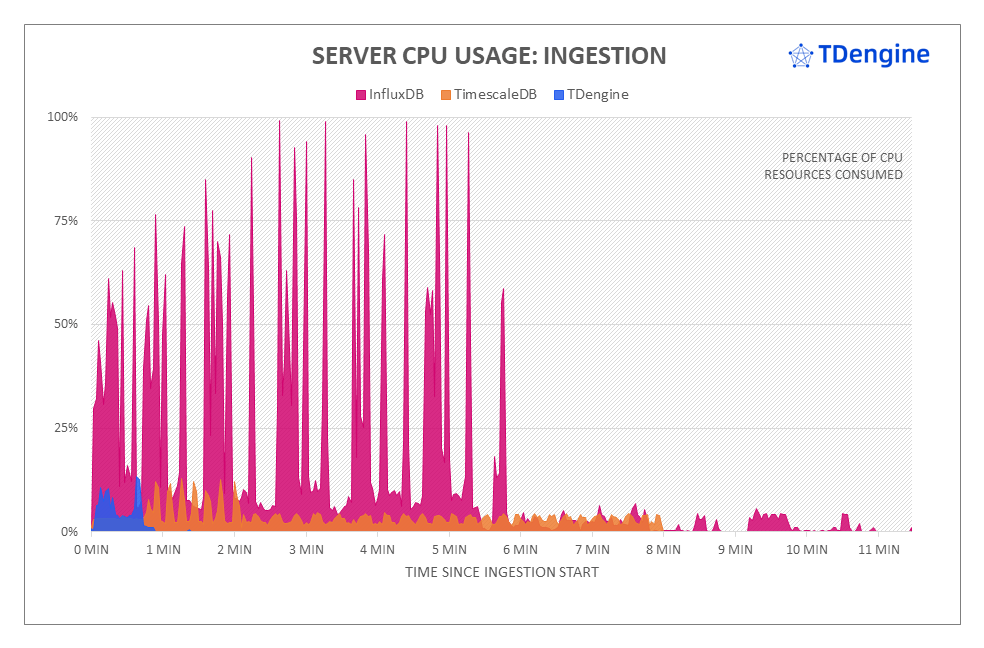
Query Performance
As performance can differ based on a number of factors, the TSBS framework covers a wide range of query types. TDengine provided the fastest query response across all scenarios, confirming that organizations dependent on real-time analytics are best served with this purpose-built platform.
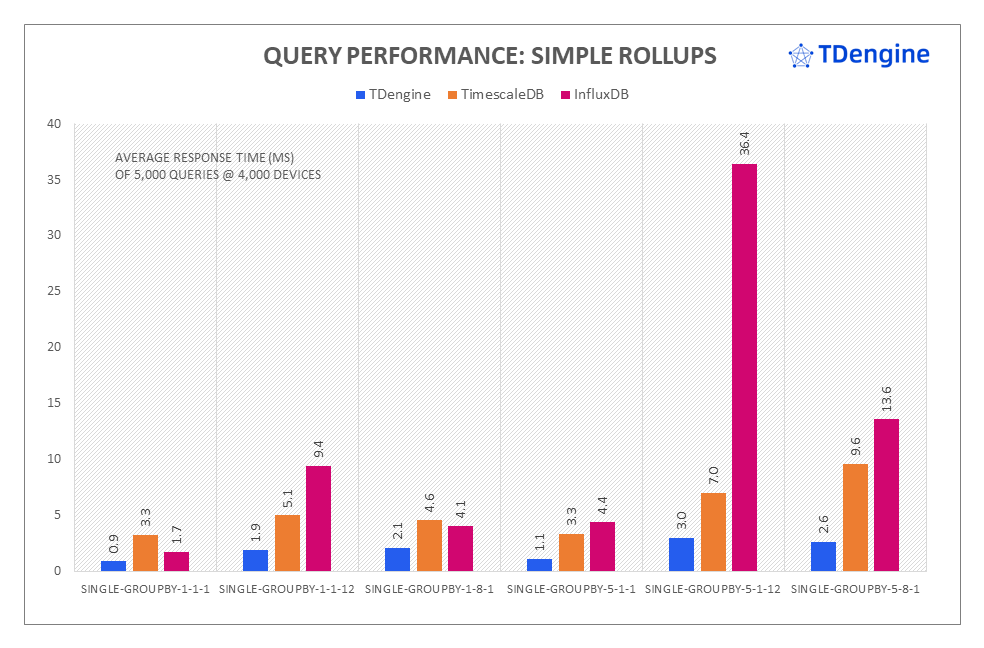
More complex queries allowed TDengine to show off its processing power, reaching 24.3 times the performance of TimescaleDB and 34.3 times the performance of InfluxDB in the most challenging scenario: double-groupby-all. This demonstrates that TDengine is best prepared to handle the most performance-intensive queries without slowing down.
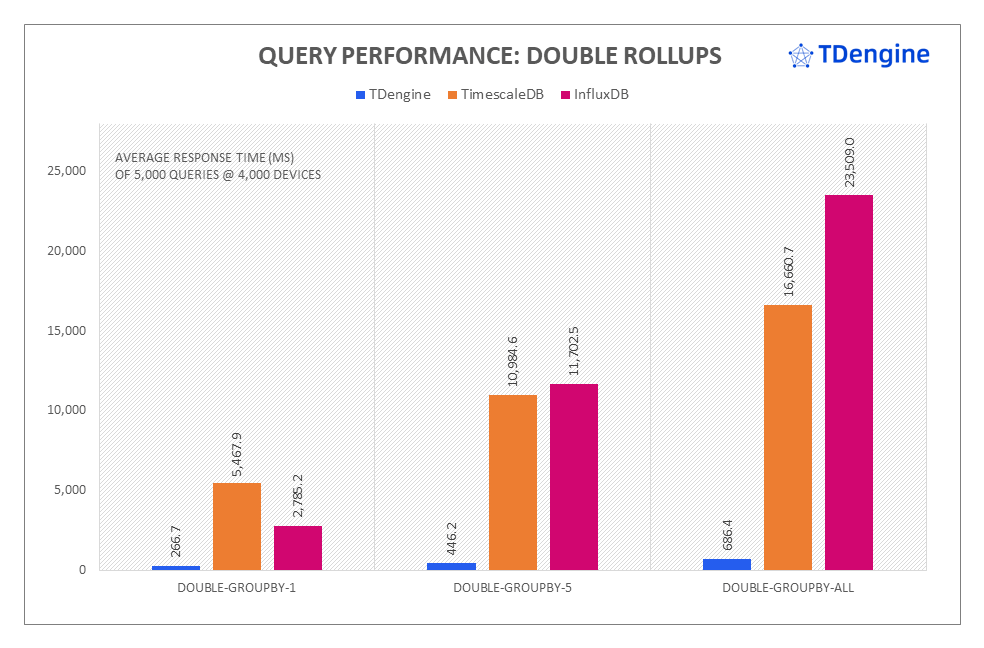
The performance of TDengine in the other TSBS query categories was as follows:
- Aggregates: Up to 6.9x faster than InfluxDB and 6.1x faster than TimescaleDB
- Thresholds: Up to 15.1x faster than InfluxDB and 1.9x faster than TimescaleDB
- Complex queries: Up to 21.1x faster than InfluxDB and 8.4x faster than TimescaleDB
Download the full report to see all of the query performance test data.
Disk Storage
In smaller-scale scenarios, TDengine and InfluxDB took up a similar amount of disk space. When the datasets increased to one million or ten million devices, however, the benefits of TDengine’s storage design and architecture came into play; for large-scale datasets, TDengine uses less than one-fourth the storage resources that InfluxDB requires.
TimescaleDB on the other hand, had a significantly higher disk footprint across all scenarios. The clearest example was in the ten million device scenario, where data processed by TimescaleDB occupied more than 26 times the disk space used by TDengine.
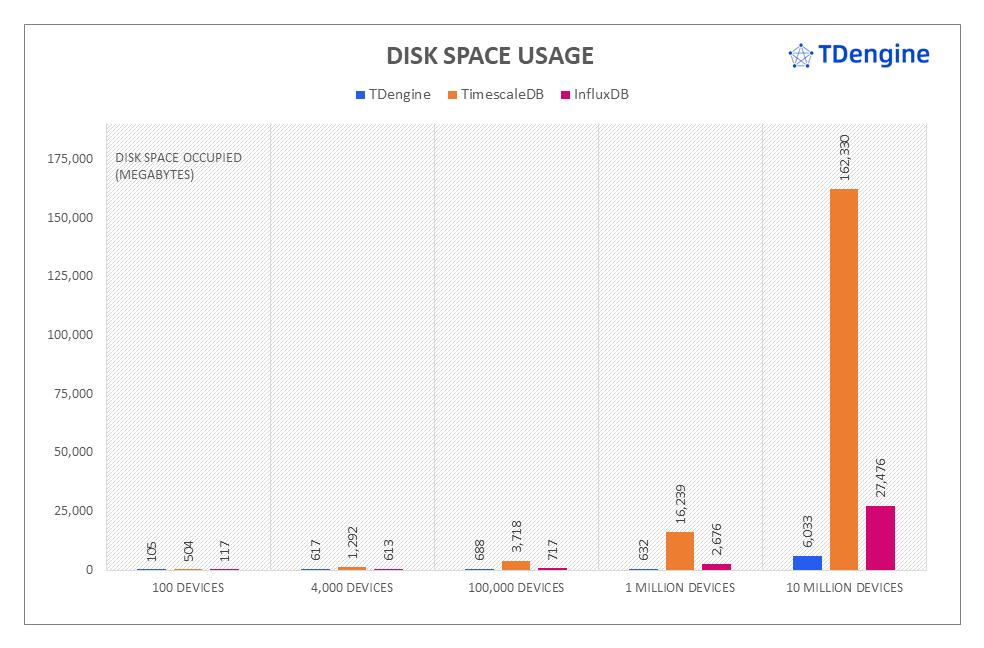
Conclusion
Across all key test metrics for ingestion, compression, and querying, TDengine clearly emerges as the highest-performing time series database.
- Ingestion: TDengine writes the test data between 1.5 to 6.7 times faster than TimescaleDB, and 3.0 to 10.6 times faster than InfluxDB, with significantly lower CPU overhead.
- Compression: Due to its efficient data storage and compression features, TDengine consumes 26.9 times less disk space than TimescaleDB, and 4.5 times less than InfluxDB.
- Queries: TDengine has the fastest query response time across all scenarios. For the most complex query type, TDengine responds 28.6 times faster than TimescaleDB and a whopping 37.0 times faster than InfluxDB.
Purpose-Built Design
Unlike all-purpose databases like MySQL or PostgreSQL (which is used by TimescaleDB), TDengine was designed from the ground up to simplify and scale time series data management. The platform’s innovative storage engine makes full use of the unique characteristics of time series data, with novel concepts like a single table for each data collection point, which enables better ingestion, and data compression, and supertables, which speed up aggregation operations.
Best in Class TSDB
The performance advantages shown by this evaluation indicate that TDengine excels at time-series data processing, especially with larger datasets and more complex queries. TDengine also requires fewer resources, significantly reducing the TCO of data operations. These advantages, combined with its comprehensive feature set and ease of use, make TDengine the best option for growing enterprises to scale their data pipelines.