Ahead of the Curve: Solve Problems Before They Happen
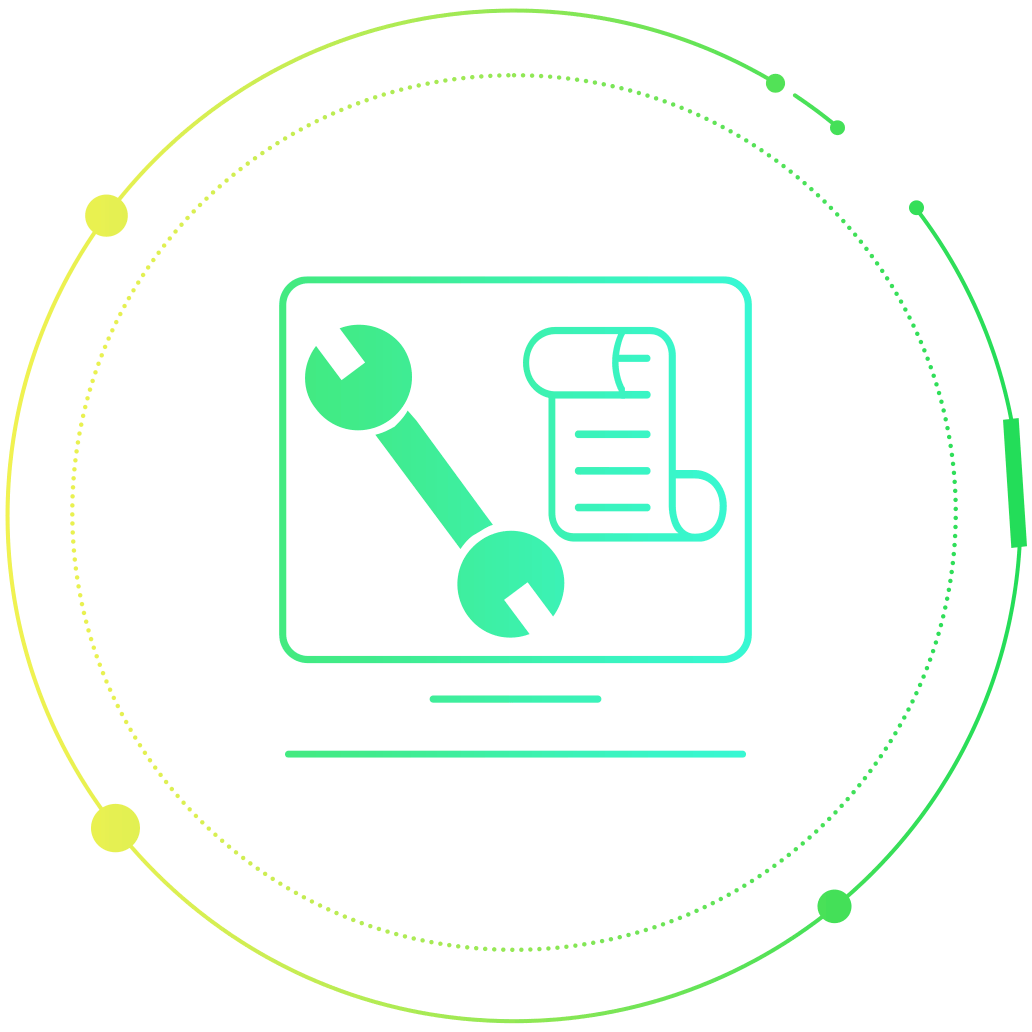
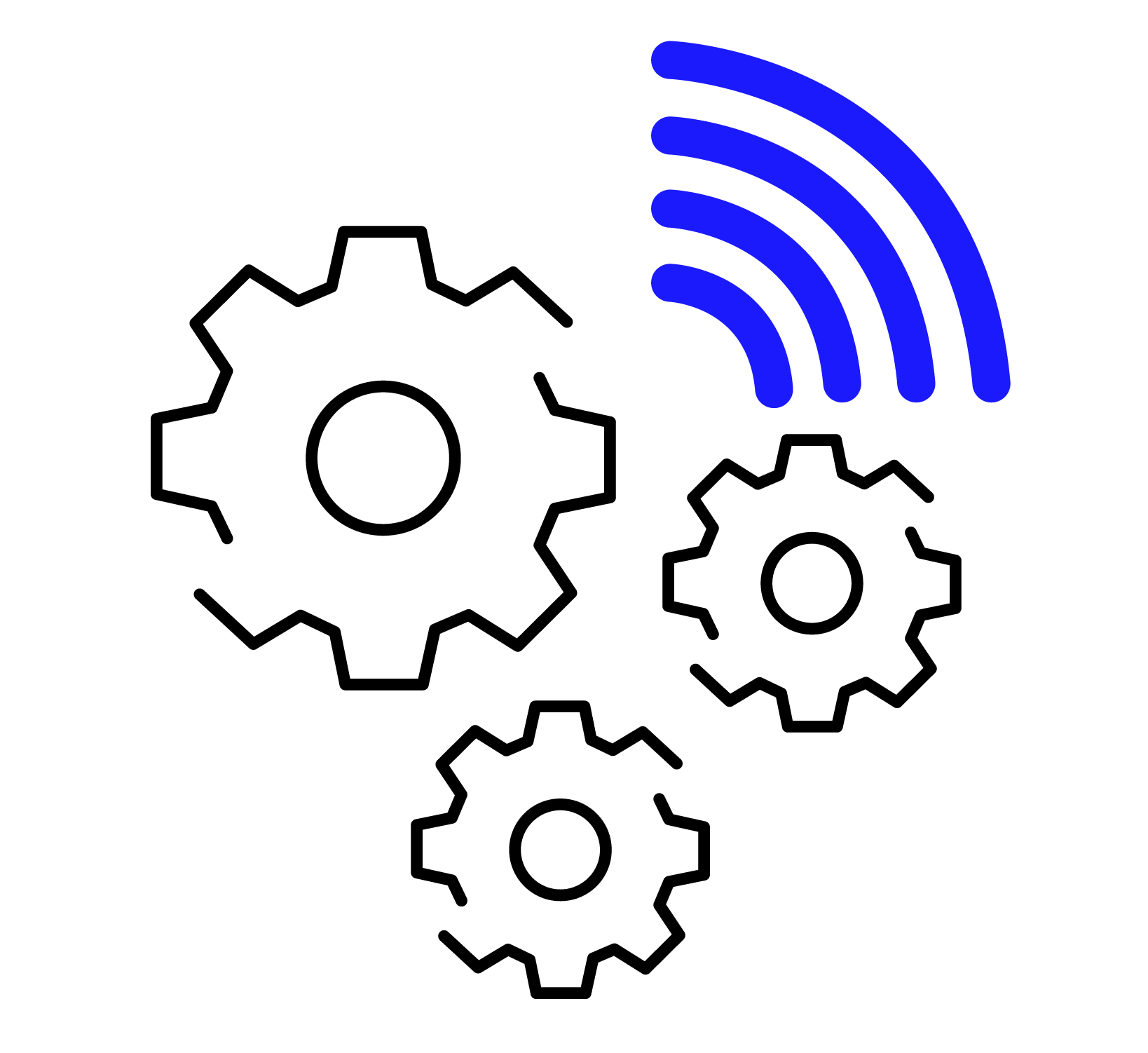
Anticipate issues and schedule maintenance before a breakdown occurs, reducing downtime and extending the lifespan of machinery.
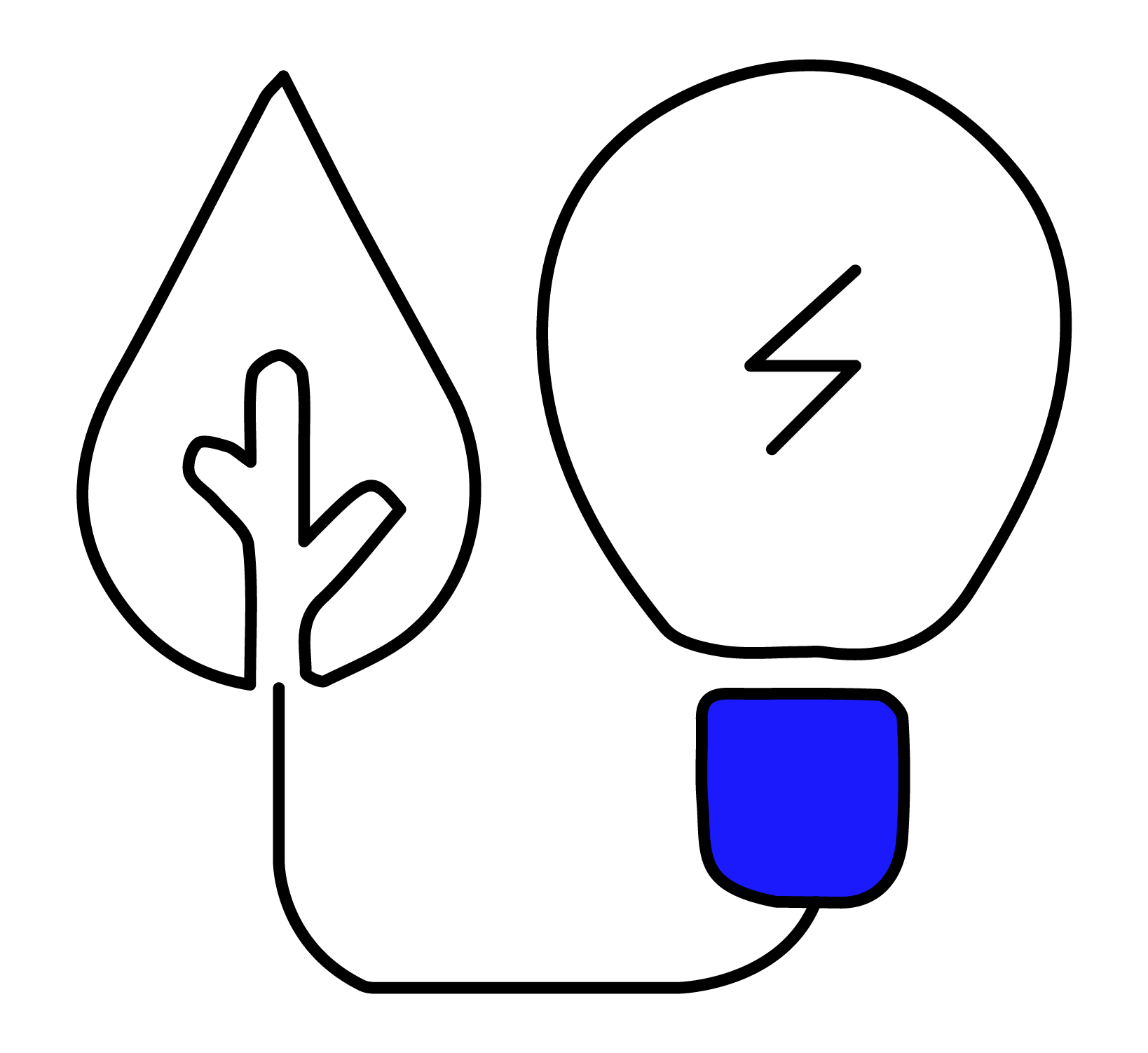
Monitor asset performance in real-time to identify early signs of degradation, optimize energy output, and reduce downtime across wind, solar, and hydropower assets.
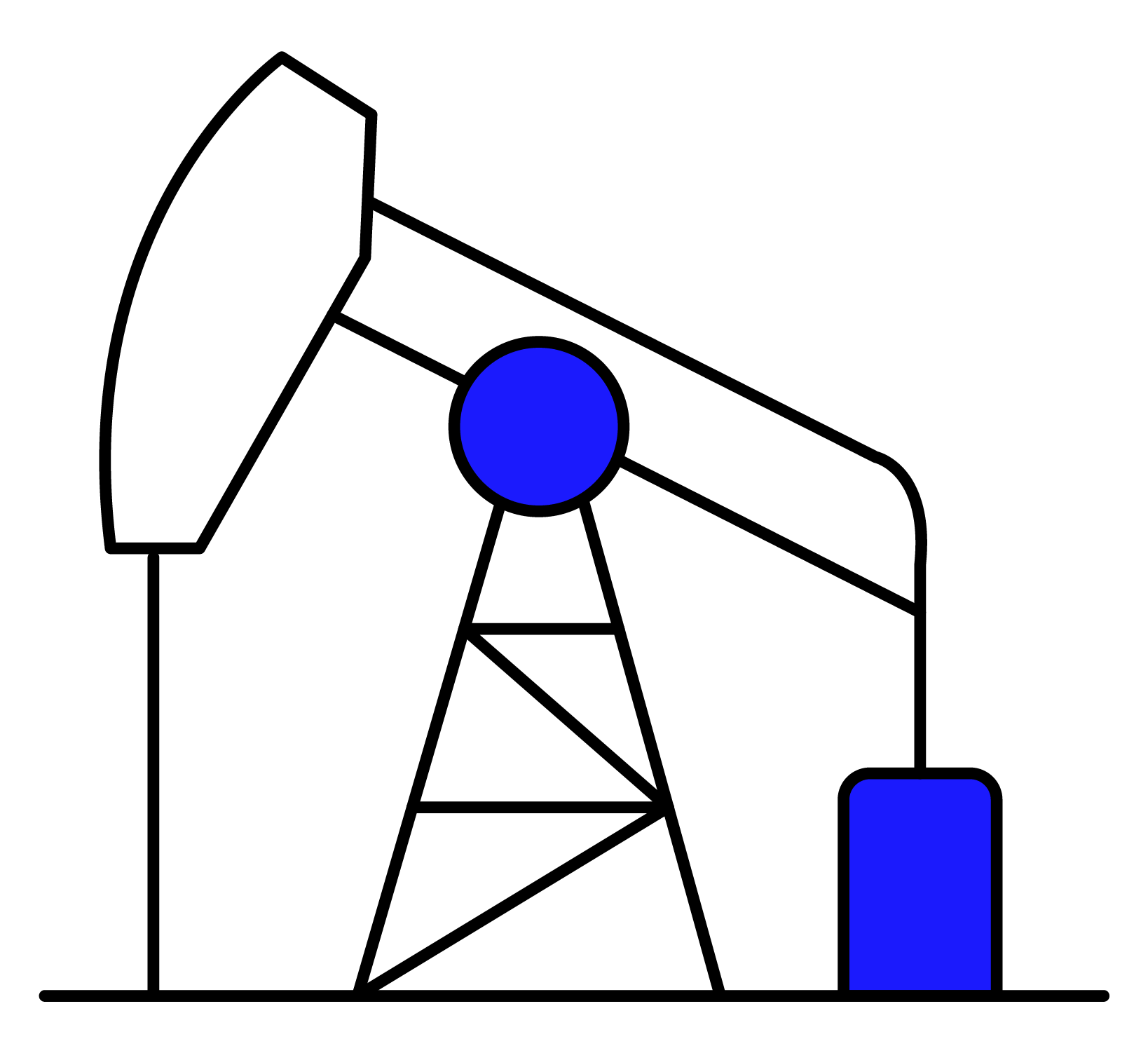
Leverage real-time data to detect anomalies, prevent critical equipment failures, and ensure safe, efficient operations in upstream, midstream, and downstream assets.
Challenges of Predictive Maintenance
Industrial assets often have thousands of sensors generating data at very high frequencies.
Years of historical sensor data must be stored and analyzed to build accurate predictive models.
Anomaly detection and predictive analytics must run continuously on streaming sensor data in real time.
Closed data systems are difficult to integrate with BI, visualization, and emerging AI/ML tools.
How TDengine Can Help
Ingest millions of sensor data points per second across billions of time series with high performance.
Enable cost-effective storage of historical sensor data with high compression and tiered storage.
Run computations like anomaly detection directly on streaming data and obtain results in real time.
Avoid vendor lock-in with seamless integration with a wide variety of third-party tools and components.
What Our Customers Say
Electric Motors
- Predictive maintenance platform with over 5,000 connected devices collecting metrics every second
- Built-in data subscription enables a warning and alarm system without reliance on custom code or third-party solutions
- Built-in caching functionality supports real-time dashboards with dynamic refreshing of the latest metrics
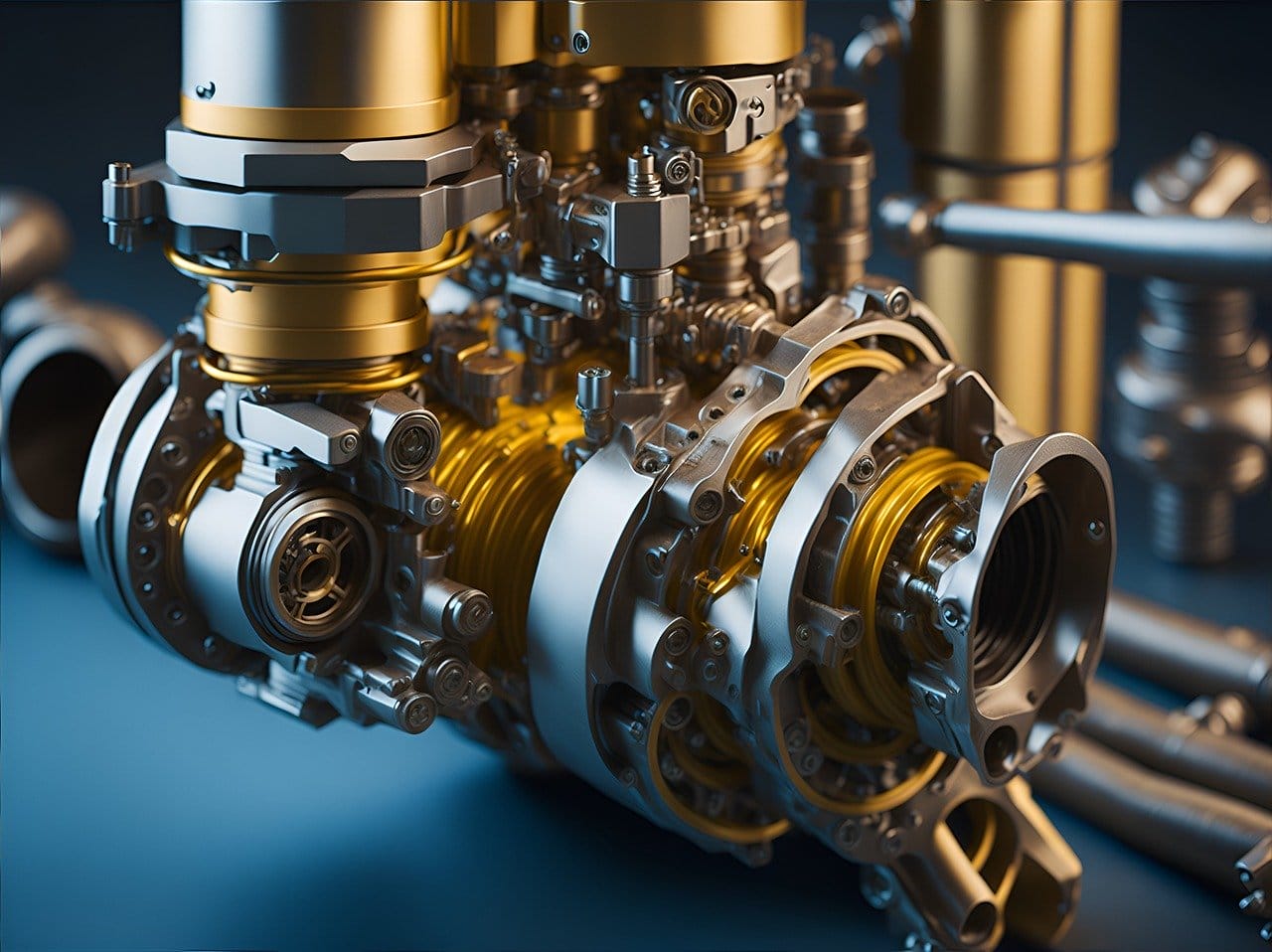
A supplier of electric motors for applications such as industrial machinery, air conditioners, and elevators developed an IoT-based platform to monitor and maintain its products. After replacing their relational database with TDengine, data is stored with greater granularity, providing more precise data support for intelligent diagnostics and analytics on the platform.
Power Plant Operations
- High-performance ingestion and aggregation query functions provide millisecond-level response to monitor power stations effectively
- Low resource usage suitable for limited hardware at power plants
- Data storage requirements approximately one-third of InfluxDB
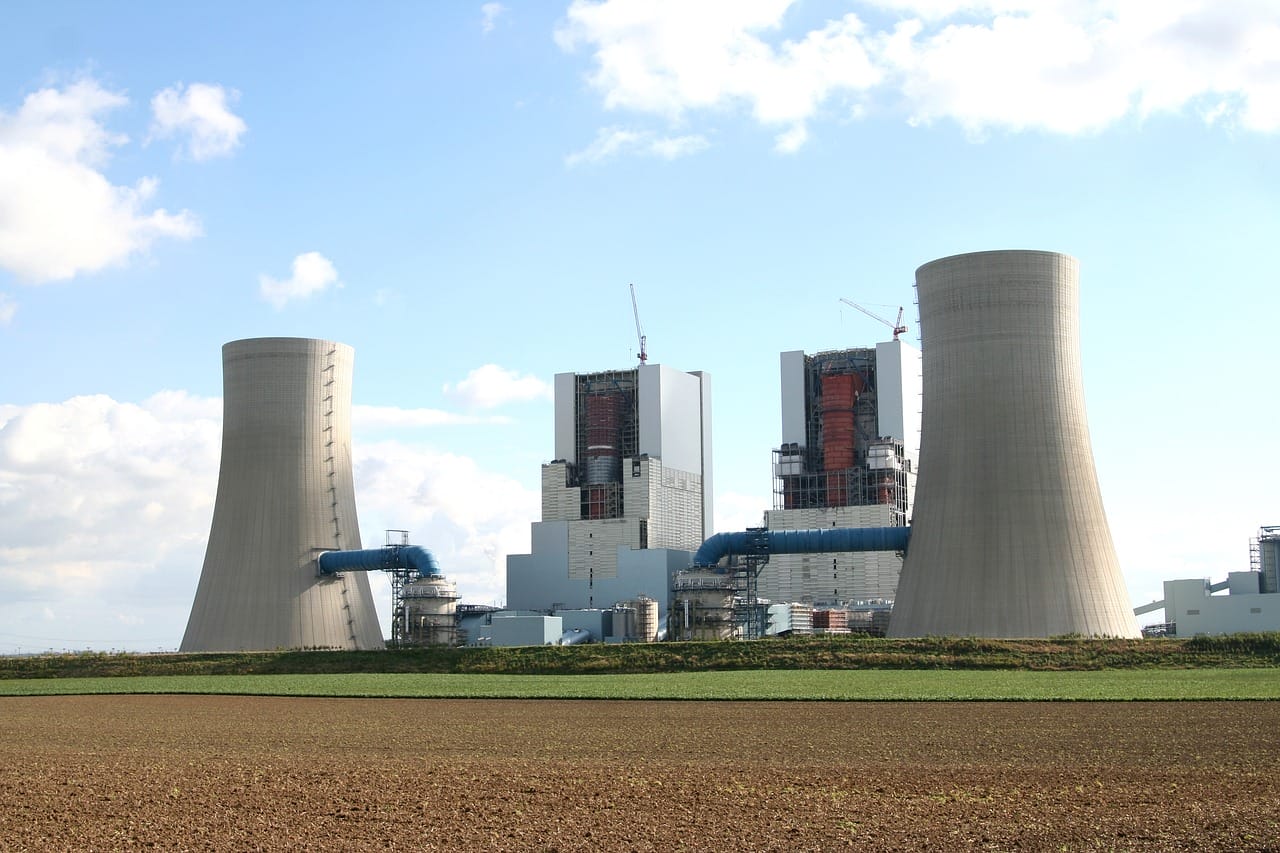
A power plant operator was deploying a monitoring system across many sites. However, the servers at each site had limited hardware specifications. TDengine was selected as a resource-efficient solution for enabling data analysis and operations optimization.