Ever since I started focusing on industrial data infrastructure, my goal has been to help customers accelerate digital transformation and make industrial data accessible, valuable, and affordable for everyone. That’s what has motivated me to develop TDengine, the next generation data historian. But when I speak with industry experts and customers, I often find that there is some uncertainty about the definition of the next generation data historian and why it’s a necessary step forward for industrial data.
History of the Historian
The traditional data historian is familiar to many industrial enterprises and has long been an essential part of their data infrastructure. The role of the historian, according to Gartner, is to “capture the output of operational technology systems, manage its life cycle, and provide access for performing some levels of data analysis.” Data historians commonly found in industrial sites today include PI System and Wonderware Historian, both originally designed in the 1980s.
While these systems were revolutionary in their time, they are not always sufficient for the business requirements of industry today. Traditional historians excel at collecting data for the individual sites in which they are deployed, but lack capabilities for global analytics, which is becoming increasingly important for optimizing efficiency. Likewise, traditional historians cannot easily share data with other systems; even today, some operators are forced to send Excel files by email when they want to provide other teams access to their operational data. And traditional historians are not designed for the cloud, holding back digital transformation plans that involve cloud migration and centralization.
As industry searches for new methods to improve operational efficiency and unlock more value from data, traditional historians frequently become a roadblock, because they cannot integrate with emerging technologies such as AI. These historians are closed systems that were never intended to support interoperability with third-party products, and their built-in analytics have long fallen behind the specialized products developed by market-leading companies.
What Should a Data Historian Do?
Typically, a traditional data historian consists of a time-series database and related applications for industrial data storage and processing. However, the scope of the historian is not always clearly defined. Apart from a time-series data processing engine and connectors for data protocols, the specific features provided by each historian may differ by vendor. Traditional historians may include some form of visualization, analytics, or asset management, for example, as built-in components. Vendors typically design their products to provide the basic features that they believe customers need for processing their operational data.
TDengine, however, does not include these additional components. In fact, people often ask me, “How can you call your product a next generation data historian when it doesn’t have feature parity with traditional historians?” But my answer is that a next generation historian does not and in fact must not provide all features of traditional historians. In the industrial data architecture of the future, these features are provided by the ecosystem, not by the historian itself. The historians of the next generation like TDengine must focus on their core competency of processing and storing time-series data along with its contextualization.
The real difference between a traditional historian and a next generation historian is simple: traditional historians are closed, monolithic systems, and next generation historians are open, composable systems.
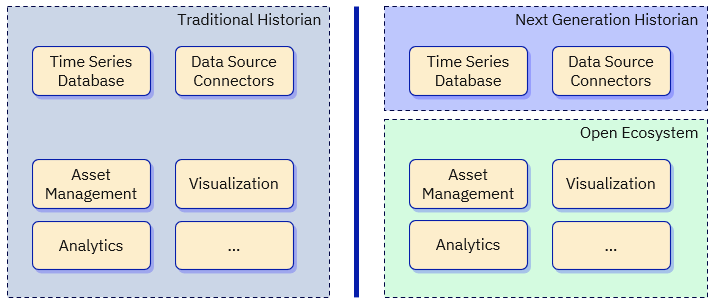
With traditional historians, all features are included in a single product or suite. You get the features and capabilities that the vendor wants you to have — no less and no more. On one hand, this can offer a simpler procurement and deployment process. But there are numerous drawbacks to the customer: most importantly, by storing your data in a closed system, you are locked to your vendor and no longer have the option of easily migrating to or testing other products. And while your historian may include components like visualization, these are typically inferior to components designed by companies that specialize in these areas.
Next generation historians, however, include only core features — the time-series database and data connectors. To obtain other features, you integrate products into your infrastructure based on your specific business needs and use cases, composing the system that’s best for your organization. In this open model, you can select the optimal components for each aspect of your system instead of relying on a single vendor to be an expert in every field. You never pay for features you don’t need, and you never miss out on features you need simply because your vendor doesn’t offer them.
In other words, TDengine empowers customers to choose best-in-class products for every single component of their infrastructure, centered around the best-in-class time-series database that we provide. We ensure that we support integration with these products and offer standard interfaces and connectors. Traditional historians’ customers, on the other hand, have no choice but to use their vendor’s solutions or waste valuable resources on custom connectors.
The Next Step Forward
For existing customers, especially in brownfield deployments, it’s true that a next generation data historian cannot replace a traditional historian. Instead, the next generation historian is deployed on top of the traditional historian to extend its capabilities and unlock the customer from the restrictions of their traditional historian vendor.
At new customers and in greenfield deployments, the traditional historian is no longer needed, as all necessary features are provided by the next generation historian and its rich ecosystem. PLCs communicate with the next generation historian over modern protocols like MQTT and OPC UA. The strong integration capabilities of next generation data historians mean that a single deployment can store and process data from both greenfield and brownfield deployments if needed.
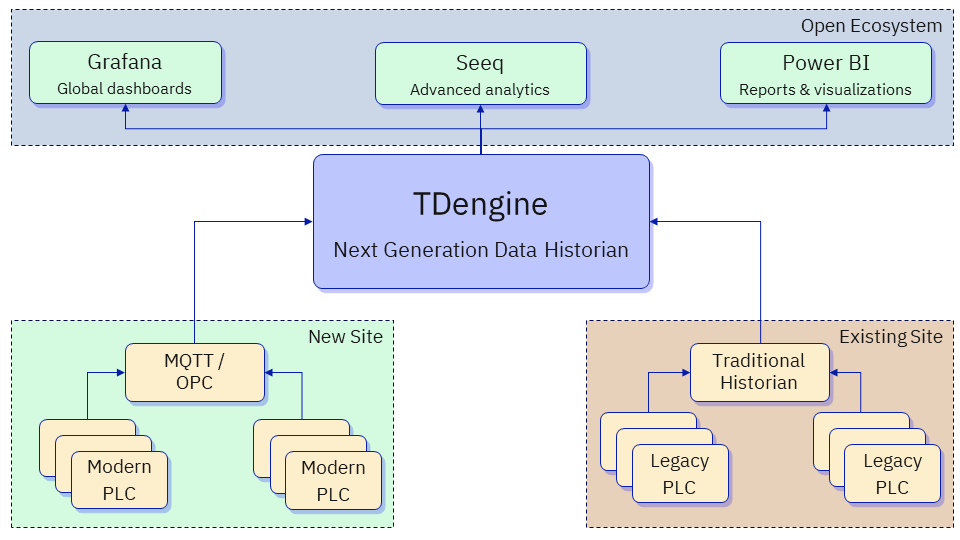
From this we can see that the next generation data historian is not a replacement for the traditional data historian, but the next step in its evolution. Modern use cases like predictive maintenance, global analytics, and digital twins need a modern data infrastructure that the historians of the previous century cannot support. Before you sign your next contract with vendors that lock you in to their closed legacy systems and deny you the freedom to use your data how you want, I encourage you to give TDengine a try and experience for yourself a future-ready industrial data platform that makes your data accessible and affordable.