Company Overview
Nevados Engineering is a California-based company that helps solar project developers and contractors to simplify the design, installation, and operation of their solar power plants. With the overarching goal of making solar a cost-competitive source of clean energy from all angles, their products increase location site options, eliminate major costs of construction, and maximize power generation.
Nevados produces a single-axis solar tracker adopted for non-flat terrain that is deployed at utility-scale projects across the United States. To support the fully autonomous operation of solar power plants, their software controls and optimizes trackers on an individual row-by-row basis in real time. Their trackers collect moment-by-moment changes in weather conditions and detailed current generation data to produce a reliable solution that enables optimal energy production.
Case Background
The previous time-series data stack at Nevados consisted of AWS IoT Core for device interconnection and Telegraf for data collection, with Amazon DynamoDB running on top as their database solution. DynamoDB, a key-value NoSQL database, was easy to get started, but its limitations became evident as Nevados completed more projects and collected more data.
Considering the nature of the time-series data that their products generated, a key-value store like DynamoDB was not able to perform acceptably as the size of their data set increased. In addition, they found DynamoDB hard to query compared with SQL databases and had difficulty accessing the data they needed when they needed it. Nevados realized that, for a growing business like itself, the solution was to replace its current database with a data historian purpose-built for industrial scenarios.
Product Selection
Having decided to deploy a data historian, Nevados compared several of the leading products on the market to determine which would be the best fit for their use case. They were highly impressed by the query performance of TDengine and its support for standard SQL. Furthermore, the distributed architecture of TDengine 3.0 would be suitable for their plans for future expansion.
Nevados was hesitant at first to try TDengine Cloud, which was newly released when this project started. Compared with the time-series database InfluxDB, TDengine had a smaller community and less comprehensive documentation. Nevados wondered whether they would have the resources needed to solve any problems that may occur. In addition, with TDengine Cloud being a new product, not all features and capabilities were fully in place from the beginning.
After working with the TDengine Team, however, both of their concerns were alleviated. First, the personal level of support offered by team members enabled quick resolution of the issues that they encountered. As a valued TDengine Cloud customer, Nevados communicated directly with the product and development teams and brought up their questions and concerns, being assured that TDengine Team members would address them as soon as they could.
Second, Nevados found the pace of innovation at TDengine to be extremely fast. In addition to addressing bugs, the development team at TDengine has worked tirelessly to improve the reliability and performance of the product. Nevados has been delighted on many occasions to find that a hotfix can be provided in a short time frame and that new features are constantly being added to the product.
In particular, with the data sharing feature of TDengine Cloud, Nevados can apply fine-grained user and data sharing privileges, which has greatly improved their overall experience and streamlined operations related to database administration. The enhanced user management system was prioritized on Nevados’s request and the entire development process was completed in 2 months.
Project Results
After deciding on TDengine as their data historian, Nevados adjusted their stack such that data being collected by Telegraf was written to a TDengine Cloud instance.
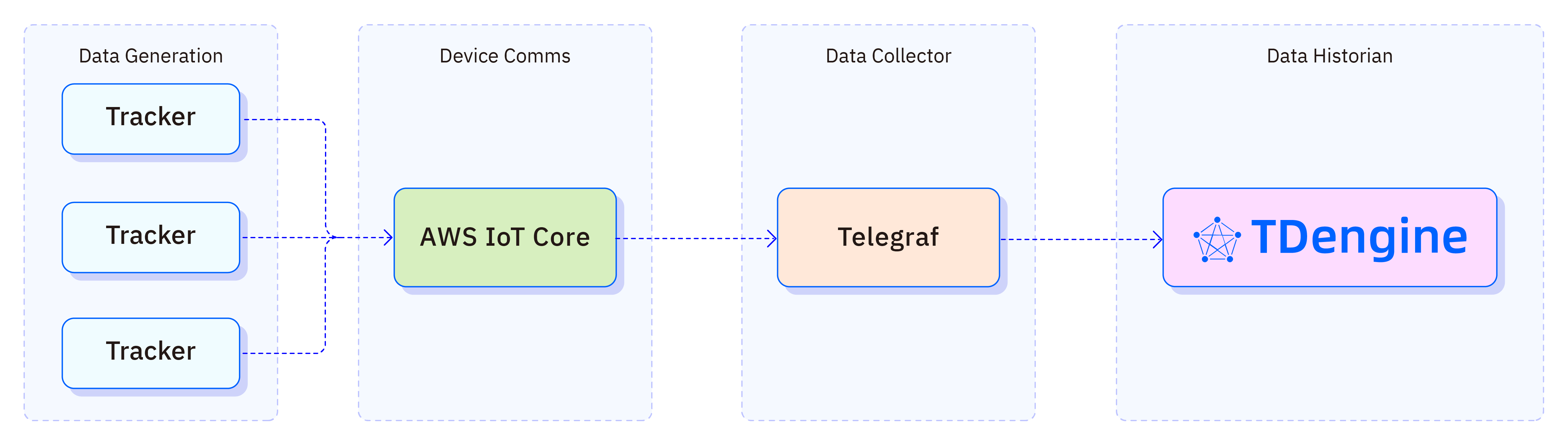
As TDengine supports data ingestion from Telegraf, Nevados was able to reconfigure their existing stack to use TDengine without changing the underlying components. The Nevados team was impressed by the speed at which TDengine processed their queries compared with other solutions. They have had a good experience using the TDengine Data Explorer to develop their queries before adding them to Grafana for dashboard prototyping and ad hoc analysis.
At present, Nevados has already migrated all the data from their previous solution to TDengine. Going forward, they plan to take advantage of the distributed architecture of TDengine and deploy TDengine Enterprise instances at project sites that replicate data from the edge to a centralized TDengine Cloud instance. Furthermore, TDengine will enable them to further simplify their data stack in the future by moving to MQTT. We at TDengine are excited to see the continuing business growth at Nevados and look forward to supporting their time-series data processing needs.