Owing to business expansion, mergers and acquisitions, vendor changes, and other factors, it’s almost inevitable that growing businesses accumulate data across a variety of disparate platforms and technologies — and equally inevitable that these platforms have difficulty communicating with each other. In fact, even when different sites use the same data platform, it’s often still difficult to get cross-site data in a single location for unified processing. Over time, these data silos become an increasingly large roadblock that holds back global analytics and management of operations data and prevents companies from realizing the true potential of their data assets.
Why Consolidate Your Operations Data?
Industrial equipment is generating more data than ever before, and modern operations increasingly rely on access to large-scale datasets. New techniques like predictive maintenance and remote monitoring provide greater benefit to the organization when they are based on more comprehensive cross-site information. Data scientists need access to massive amounts of high-quality data for their AI and ML applications to be effective. And decision-makers want to see global statistics in real time under a single pane of glass.
Unfortunately, many organizations still rely on manual workloads to centralize their data — to this day there are people with no choice but to email CSV files to share their data with other teams. For applications that work in real time, this process means that the data is outdated before the email has arrived. And due to the different data protocols in place in various industrial systems, even organizations that have implemented automated solutions often rely on custom-built connectors that are fragile and costly to maintain.
When the silos in an organization are broken down and all operations data is consolidated in a single repository, access to the data is improved, and the manual workloads involved in preparing and transmitting it are eliminated. Teams can then work with real-time data for faster response times and more efficient problem-solving, and also collaborate more efficiently with analysts and data scientists inside and outside the organization.
Furthermore, data consolidation enables advanced analytics that can produce new insights from industrial data to improve operational efficiency in ways previously impossible. Key stakeholders are finally empowered with comprehensive datasets and can make well-informed decisions based on accurate and relevant information.
How TDengine Can Help
TDengine is a zero-code platform that requires only minimal configuration to implement the extract, transform, and load (ETL) process for data from any source, whose data is then centralized in our purpose-built time-series database.
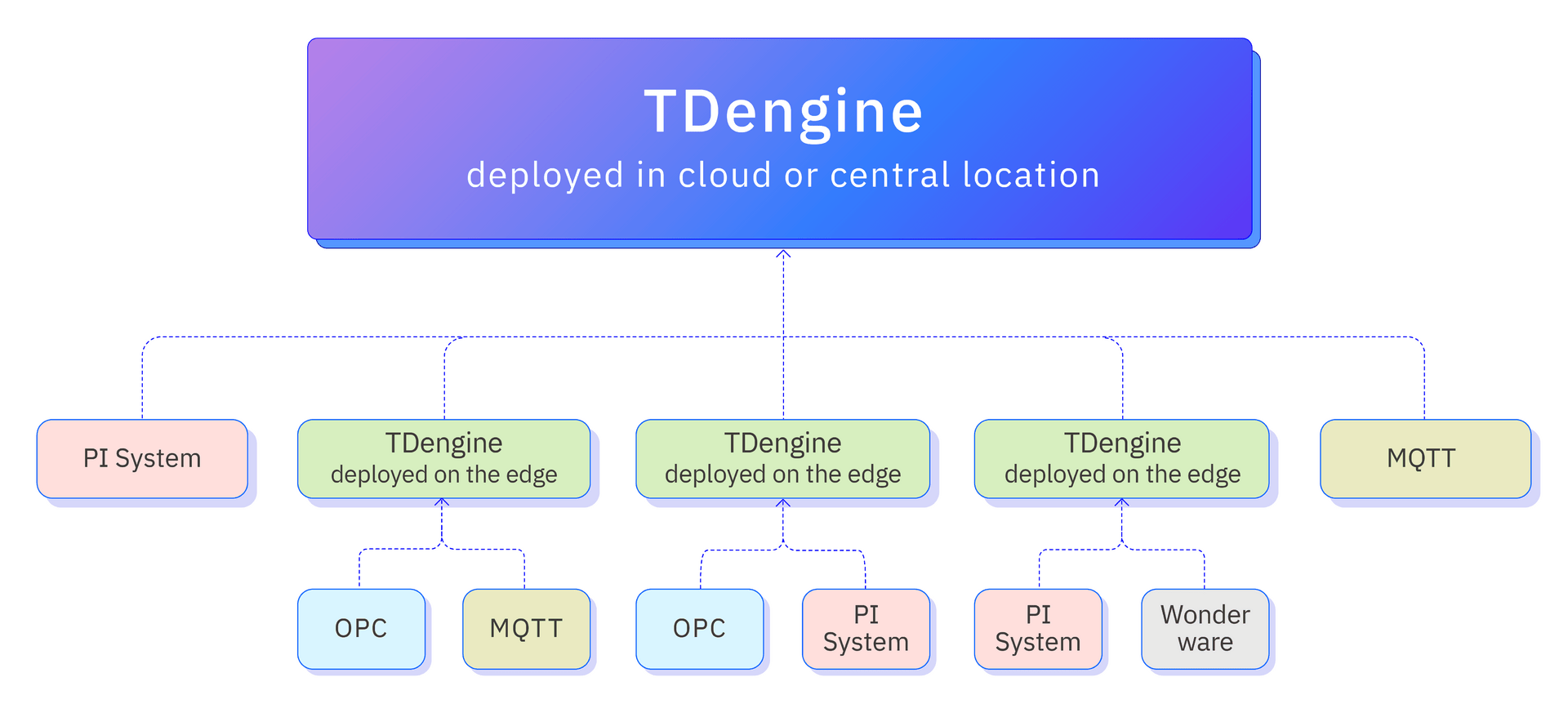
Cross-Platform Data Ingestion
With TDengine, you can easily consolidate industrial data from a wide variety of platforms into a single source of truth for your business. These include, among others:
- Modern industrial data protocols like MQTT and OPC (UA and DA)
- Data collectors like Telegraf and collectd
- Traditional data historians like PI System and AVEVA Historian
- Apache Kafka
- CSV files
After data from all your sites and platforms has been ingested into TDengine, you can easily integrate visualization and business intelligence tools such as Seeq or Power BI to build company-wide dashboards and reports or connect with AI platforms for advanced analytics. Your applications and algorithms have access to all data in real time, enabling global insights and efficiency without custom code or manual operations.
Advanced ETL
When you consolidate your data in the unified TDengine platform, you can make modifications to the data to ensure that context is preserved and business requirements are met. This process is known as extract, transform, and load (ETL). For example, consider equipment at one site that records temperature in degrees Fahrenheit, while equipment at other sites uses degrees Celsius. You can configure TDengine to transform this data to use the same unit of measurement and give your applications access to more standardized datasets.
Another common scenario is that different sites store the same metric under different names — “voltage” and “volts,” for example. You can easily configure TDengine to ingest these metrics into a single column, further consolidating your data and reducing the application development workload by handling transformation on the database side. With TDengine’s flexible and easily configurable ETL options, available in the GUI, you can transform, map, split, and parse your data so that it’s a perfect fit for your use case.